
Machine-Enabled FP&A: Unlocking Potential
14 Ιανουαρίου 2023

Machine-Enabled FP&A: Unlocking Potential

Machine-Enabled FP&A: Unlocking Potential
Algorithmic, machine-powered forecasting has risen in recent years from a nice-to-have to a foundational finance capability. Companies are increasingly investing in sophisticated forecasting tools to try to meet strategic objectives, overcome capacity constraints, and navigate changing market conditions quickly and effectively.
At the same time, however, some users struggle to trust the produced outputs, adopt the technologies, or incorporate them into their ways of working. As a result, many companies have yet to unlock the true potential of algorithmic forecasting.
What are the barriers to successful adoption and value realization with algorithmic and machine-enabled forecasting? Addressing the following six themes can enable the successful rollout of algorithmic forecasting in financial planning and analysis (FP&A) so companies can leverage more integrated and efficient processes to accomplish strategic objectives and drive long-term adoption.Decision-making influence. Leveraging a behavioral-backed approach can help alleviate reluctance around algorithmic technology by aligning user motivators and incentives.Operating model alignment. Removing silos between algorithmic forecast modelers and consumers by establishing a common language can translate data science-driven output into meaningful, transparent insights.
Algorithmic, machine-powered forecasting has risen in recent years from a nice-to-have to a foundational finance capability. Companies are increasingly investing in sophisticated forecasting tools to try to meet strategic objectives, overcome capacity constraints, and navigate changing market conditions quickly and effectively.
At the same time, however, some users struggle to trust the produced outputs, adopt the technologies, or incorporate them into their ways of working. As a result, many companies have yet to unlock the true potential of algorithmic forecasting.
What are the barriers to successful adoption and value realization with algorithmic and machine-enabled forecasting? Addressing the following six themes can enable the successful rollout of algorithmic forecasting in financial planning and analysis (FP&A) so companies can leverage more integrated and efficient processes to accomplish strategic objectives and drive long-term adoption.Decision-making influence. Leveraging a behavioral-backed approach can help alleviate reluctance around algorithmic technology by aligning user motivators and incentives.Operating model alignment. Removing silos between algorithmic forecast modelers and consumers by establishing a common language can translate data science-driven output into meaningful, transparent insights.
Algorithmic, machine-powered forecasting has risen in recent years from a nice-to-have to a foundational finance capability. Companies are increasingly investing in sophisticated forecasting tools to try to meet strategic objectives, overcome capacity constraints, and navigate changing market conditions quickly and effectively.
At the same time, however, some users struggle to trust the produced outputs, adopt the technologies, or incorporate them into their ways of working. As a result, many companies have yet to unlock the true potential of algorithmic forecasting.
What are the barriers to successful adoption and value realization with algorithmic and machine-enabled forecasting? Addressing the following six themes can enable the successful rollout of algorithmic forecasting in financial planning and analysis (FP&A) so companies can leverage more integrated and efficient processes to accomplish strategic objectives and drive long-term adoption.Decision-making influence. Leveraging a behavioral-backed approach can help alleviate reluctance around algorithmic technology by aligning user motivators and incentives.Operating model alignment. Removing silos between algorithmic forecast modelers and consumers by establishing a common language can translate data science-driven output into meaningful, transparent insights.
Human-Centered Design
Human-Centered Design
Human-Centered Design
The first four themes above address user experience and adoption issues vs. technology and data considerations—how to integrate algorithmic forecasting into new ways of working and the most salient, people-centric challenges.
Human-centered design focuses on taking a holistic approach to developing a forecasting capability by incorporating individual requirements of the key user groups and the interactions across them. In this approach, user groups, also known as personas, are not defined by their function or business unit but by their business objectives. For example, while a vice president and an analyst may both sit in FP&A, their goals will be different. The former will need the means to toggle effectively between scenarios that model different business decisions, while the latter will seek the functionality to build those scenarios, assign values, set relevant thresholds, and complete their work accordingly.
For that reason, promoting successful adoption of a solution depends on asking two vital questions: Did we account for the right user groups or personas when designing the capability, or were the personas defined too broadly? And did we successfully connect the dots between each user group to create a comprehensive solution?
Adoption challenges could signal a missed or misaligned persona during the initial design. It’s critical to define meaningful personas through comprehensive discovery and research. Doing so involves working closely with users to understand their role within the forecast process, intended outputs, and interdependencies with other groups. The personas then become the basis of the solution design, creating a unique experience for each user group while addressing any cross-persona interactions.
A persona-agnostic design approach for an algorithmic forecasting solution can lead to an overly complex user interface. If every feature and function is available to all, users may not be able to navigate the solution easily and may be inclined to prepare their forecast outside of it. As a result, aggregation can’t be conducted systematically without the proper inputs. Even if only one stakeholder group is directly affected, rejection of the capability could cause a chain reaction, leading to widespread failed adoption.
Another consequence could be a lack of governance around sequencing, handoffs, and workflow visibility, elements that are critical to collaboration and aggregation within the forecast process. For example, without proper user groups in the workflow, tax rates could be applied to an aggregate forecast while adjustments are made at a lower level, resulting in unnecessary reconciliation issues and the loss of a key feature of the forecasting process. As algorithmic forecasting introduces a new way of working, adding persona-based embedded workflow into a forecasting solution can also support change management and training, which aids in the adoption of the solution.
The first four themes above address user experience and adoption issues vs. technology and data considerations—how to integrate algorithmic forecasting into new ways of working and the most salient, people-centric challenges.
Human-centered design focuses on taking a holistic approach to developing a forecasting capability by incorporating individual requirements of the key user groups and the interactions across them. In this approach, user groups, also known as personas, are not defined by their function or business unit but by their business objectives. For example, while a vice president and an analyst may both sit in FP&A, their goals will be different. The former will need the means to toggle effectively between scenarios that model different business decisions, while the latter will seek the functionality to build those scenarios, assign values, set relevant thresholds, and complete their work accordingly.
For that reason, promoting successful adoption of a solution depends on asking two vital questions: Did we account for the right user groups or personas when designing the capability, or were the personas defined too broadly? And did we successfully connect the dots between each user group to create a comprehensive solution?
Adoption challenges could signal a missed or misaligned persona during the initial design. It’s critical to define meaningful personas through comprehensive discovery and research. Doing so involves working closely with users to understand their role within the forecast process, intended outputs, and interdependencies with other groups. The personas then become the basis of the solution design, creating a unique experience for each user group while addressing any cross-persona interactions.
A persona-agnostic design approach for an algorithmic forecasting solution can lead to an overly complex user interface. If every feature and function is available to all, users may not be able to navigate the solution easily and may be inclined to prepare their forecast outside of it. As a result, aggregation can’t be conducted systematically without the proper inputs. Even if only one stakeholder group is directly affected, rejection of the capability could cause a chain reaction, leading to widespread failed adoption.
Another consequence could be a lack of governance around sequencing, handoffs, and workflow visibility, elements that are critical to collaboration and aggregation within the forecast process. For example, without proper user groups in the workflow, tax rates could be applied to an aggregate forecast while adjustments are made at a lower level, resulting in unnecessary reconciliation issues and the loss of a key feature of the forecasting process. As algorithmic forecasting introduces a new way of working, adding persona-based embedded workflow into a forecasting solution can also support change management and training, which aids in the adoption of the solution.
The first four themes above address user experience and adoption issues vs. technology and data considerations—how to integrate algorithmic forecasting into new ways of working and the most salient, people-centric challenges.
Human-centered design focuses on taking a holistic approach to developing a forecasting capability by incorporating individual requirements of the key user groups and the interactions across them. In this approach, user groups, also known as personas, are not defined by their function or business unit but by their business objectives. For example, while a vice president and an analyst may both sit in FP&A, their goals will be different. The former will need the means to toggle effectively between scenarios that model different business decisions, while the latter will seek the functionality to build those scenarios, assign values, set relevant thresholds, and complete their work accordingly.
For that reason, promoting successful adoption of a solution depends on asking two vital questions: Did we account for the right user groups or personas when designing the capability, or were the personas defined too broadly? And did we successfully connect the dots between each user group to create a comprehensive solution?
Adoption challenges could signal a missed or misaligned persona during the initial design. It’s critical to define meaningful personas through comprehensive discovery and research. Doing so involves working closely with users to understand their role within the forecast process, intended outputs, and interdependencies with other groups. The personas then become the basis of the solution design, creating a unique experience for each user group while addressing any cross-persona interactions.
A persona-agnostic design approach for an algorithmic forecasting solution can lead to an overly complex user interface. If every feature and function is available to all, users may not be able to navigate the solution easily and may be inclined to prepare their forecast outside of it. As a result, aggregation can’t be conducted systematically without the proper inputs. Even if only one stakeholder group is directly affected, rejection of the capability could cause a chain reaction, leading to widespread failed adoption.
Another consequence could be a lack of governance around sequencing, handoffs, and workflow visibility, elements that are critical to collaboration and aggregation within the forecast process. For example, without proper user groups in the workflow, tax rates could be applied to an aggregate forecast while adjustments are made at a lower level, resulting in unnecessary reconciliation issues and the loss of a key feature of the forecasting process. As algorithmic forecasting introduces a new way of working, adding persona-based embedded workflow into a forecasting solution can also support change management and training, which aids in the adoption of the solution.



Algorithmic forecasting capabilities can unlock benefits such as improved productivity—enabling a more detailed and granular forecast than before and generating more accurate outcomes without driving additional effort to produce it. But while these solutions serve as a central component of the forecasting process, they are not intended to replace the process entirely. In fact, deploying a forecasting solution presents an opportunity to rethink current processes and take advantage of the automation it enables.
However, ensuring the solution fits into an organization’s forecast approach is of critical importance. Because a top-down forecast typically doesn’t require the same granularity and complexity as a bottom-up forecast, each requires different considerations. Adoption challenges can often be traced back to a disconnect between the algorithmic solution and the broader forecast process. Introducing complex models and large datasets without the mechanisms to understand them can create a “black box” perception, whereby the machine outputs seem opaque and unexplainable.
Focusing on such elements as divisions, segments, and regions that drive most of the activity or volume for the business may be best suited for the algorithmic forecast, the better to maximize its impact while reducing the noise created by superfluous data. Considering how best to leverage the forecasting solution requires understanding the rationale behind the data collection: Are you including immaterial line items or products in the forecast? For example, are you forecasting for revenue or inconsequential meeting expenses within your time and expense line? The solution isn’t intended to consider every line item but rather to forecast the line items that matter most to business planning.
Algorithmic forecasting capabilities can unlock benefits such as improved productivity—enabling a more detailed and granular forecast than before and generating more accurate outcomes without driving additional effort to produce it. But while these solutions serve as a central component of the forecasting process, they are not intended to replace the process entirely. In fact, deploying a forecasting solution presents an opportunity to rethink current processes and take advantage of the automation it enables.
However, ensuring the solution fits into an organization’s forecast approach is of critical importance. Because a top-down forecast typically doesn’t require the same granularity and complexity as a bottom-up forecast, each requires different considerations. Adoption challenges can often be traced back to a disconnect between the algorithmic solution and the broader forecast process. Introducing complex models and large datasets without the mechanisms to understand them can create a “black box” perception, whereby the machine outputs seem opaque and unexplainable.
Focusing on such elements as divisions, segments, and regions that drive most of the activity or volume for the business may be best suited for the algorithmic forecast, the better to maximize its impact while reducing the noise created by superfluous data. Considering how best to leverage the forecasting solution requires understanding the rationale behind the data collection: Are you including immaterial line items or products in the forecast? For example, are you forecasting for revenue or inconsequential meeting expenses within your time and expense line? The solution isn’t intended to consider every line item but rather to forecast the line items that matter most to business planning.
Algorithmic forecasting capabilities can unlock benefits such as improved productivity—enabling a more detailed and granular forecast than before and generating more accurate outcomes without driving additional effort to produce it. But while these solutions serve as a central component of the forecasting process, they are not intended to replace the process entirely. In fact, deploying a forecasting solution presents an opportunity to rethink current processes and take advantage of the automation it enables.
However, ensuring the solution fits into an organization’s forecast approach is of critical importance. Because a top-down forecast typically doesn’t require the same granularity and complexity as a bottom-up forecast, each requires different considerations. Adoption challenges can often be traced back to a disconnect between the algorithmic solution and the broader forecast process. Introducing complex models and large datasets without the mechanisms to understand them can create a “black box” perception, whereby the machine outputs seem opaque and unexplainable.
Focusing on such elements as divisions, segments, and regions that drive most of the activity or volume for the business may be best suited for the algorithmic forecast, the better to maximize its impact while reducing the noise created by superfluous data. Considering how best to leverage the forecasting solution requires understanding the rationale behind the data collection: Are you including immaterial line items or products in the forecast? For example, are you forecasting for revenue or inconsequential meeting expenses within your time and expense line? The solution isn’t intended to consider every line item but rather to forecast the line items that matter most to business planning.

Διαβάστε επίσης...

21 Μαΐ 2025
Η Seven Red Lines στο Sales in Action 2025

21 Μαΐ 2025
Η Seven Red Lines στο Sales in Action 2025

21 Μαΐ 2025
Η Seven Red Lines στο Sales in Action 2025

15 Απρ 2025
Causal AI in business risk management – the Insurance paradigm

15 Απρ 2025
Causal AI in business risk management – the Insurance paradigm

15 Απρ 2025
Causal AI in business risk management – the Insurance paradigm
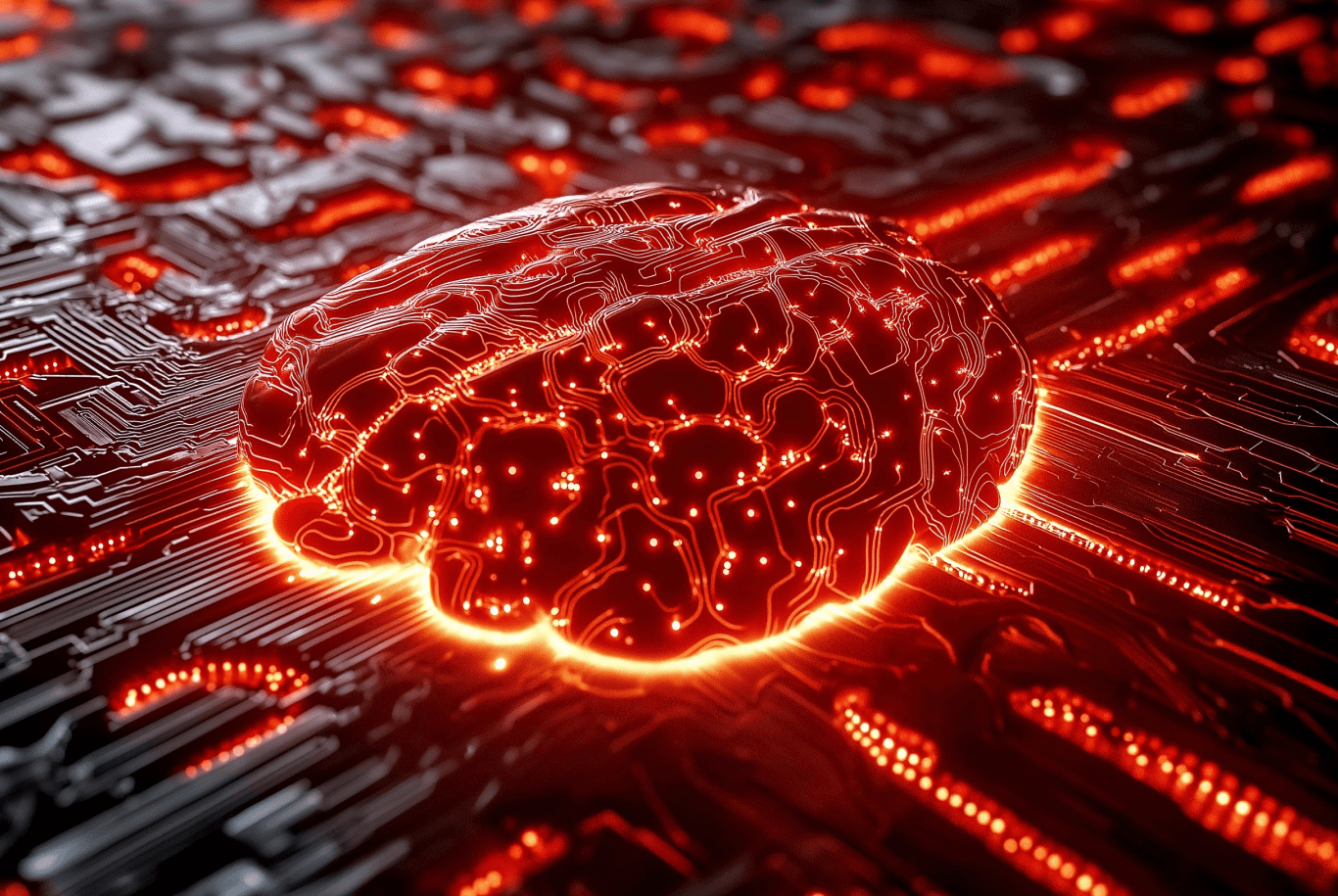
21 Νοε 2024
EU AI ACT: A comprehensive overview of the new AI regulation framework
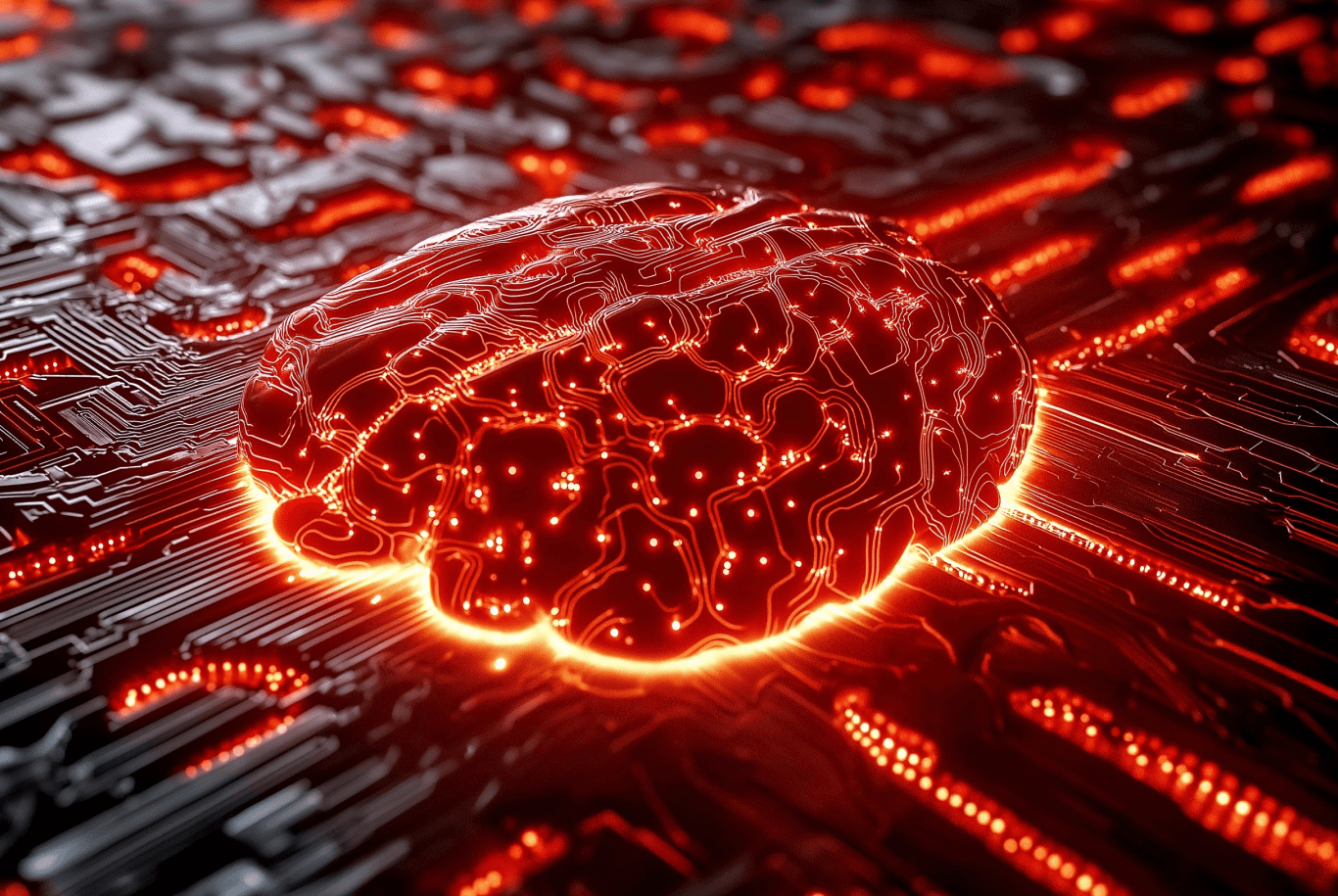
21 Νοε 2024
EU AI ACT: A comprehensive overview of the new AI regulation framework
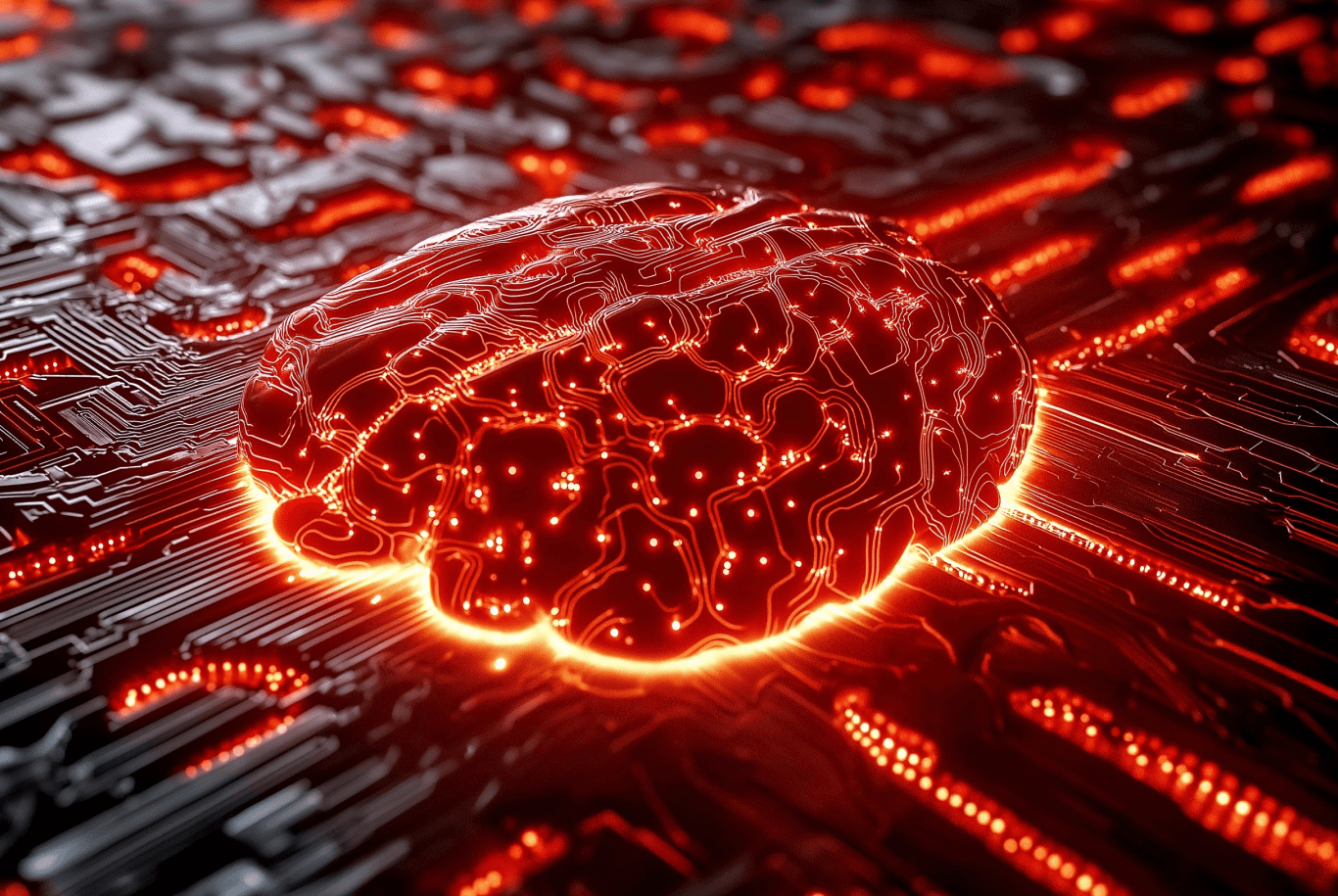
21 Νοε 2024